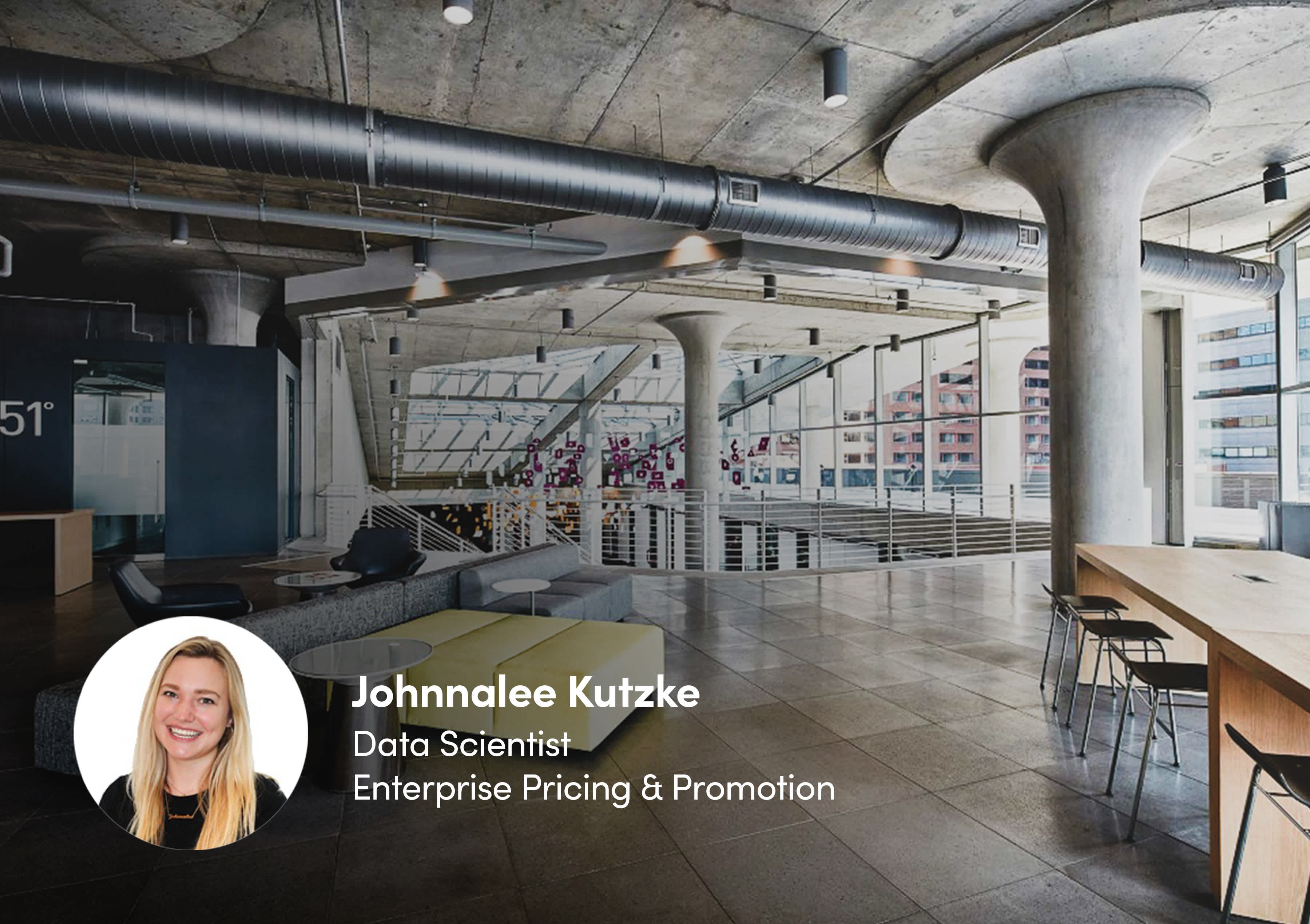
Grassroots education on the implications of gender bias
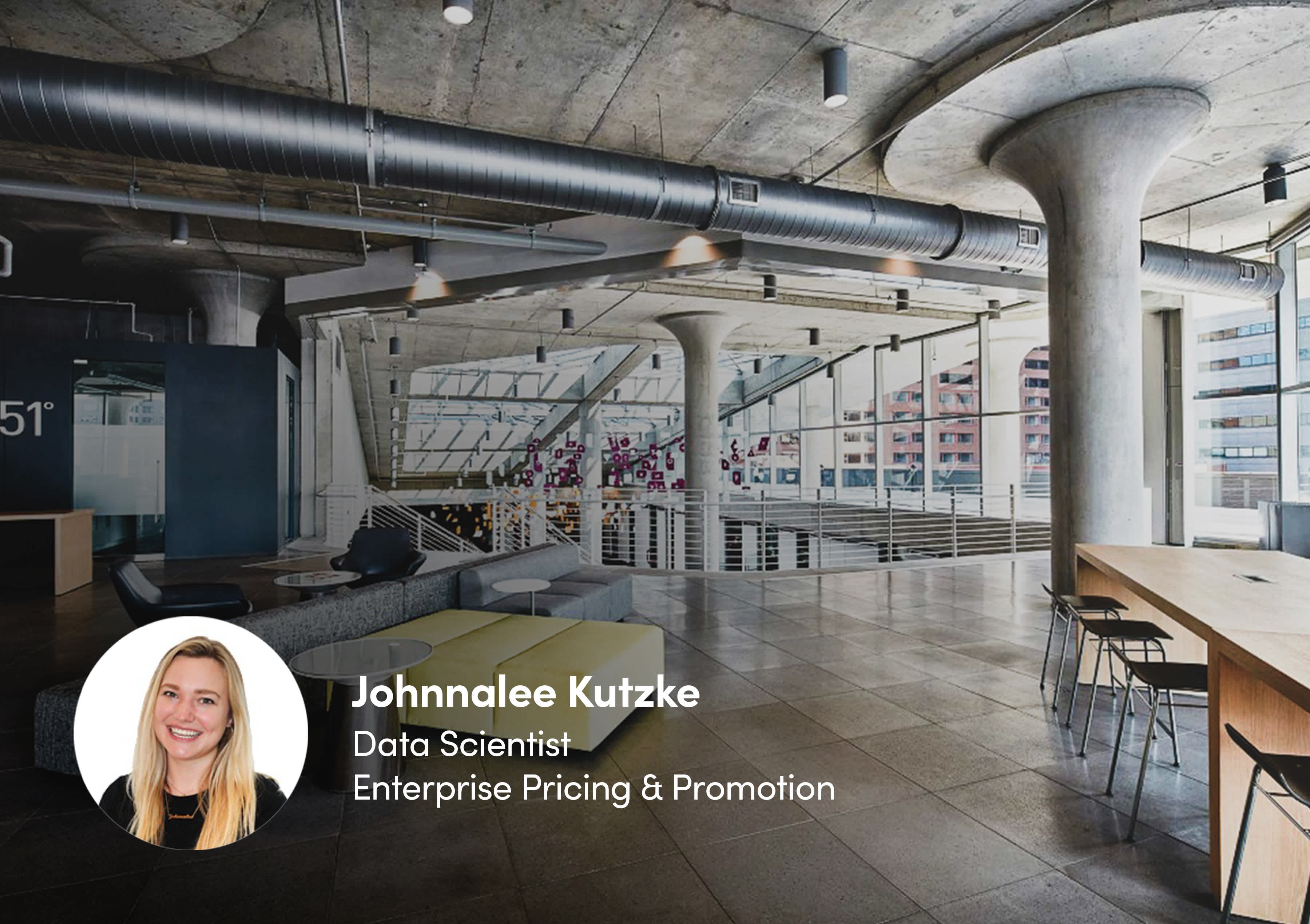
Welcome to 84.51° Data University, a series of quarterly insights for prospective and current data-science professionals.
Can you give us some background on the work that you did to educate others about gender bias?
I’ve done a couple of presentations - the first was at an internal data science technical community meeting where I pulled together a presentation on gender bias in natural language processing (NLP). I have since repurposed the presentation at a university and have also supported work exploring intersectionality of bias in other org-wide forums.
Your story is unique in that it was self-started. What called you to action?
Well, a couple of things. I am personally passionate about bias mitigation in general. This work was specifically inspired by the rise of ChatGPT and I wanted to bring awareness to some of the inherent biases in it. Second, my people leader has also been amazingly encouraging about me not only pursuing the work, but also taking time in the workday to research and create the content.
“Remediation happens in layers. As data scientists, we help business leaders understand the positioning and application of a model to ensure there is awareness of bias…"
How was your work received?
I was surprised it was so well received and my voice was amplified. I had people reaching out saying they appreciated the content and then I had other groups later reach out about doing more in the space. Those in leadership roles elevated my voice too.
I recently had the opportunity to present as part of a Black History Month lunch and learn on inclusion in AI. Intersectionality in bias is so important.
Do you have an example you feel comfortable sharing where this work has helped you or others make different decisions?
This one is small and not necessarily formal or a direct consult from the presentation. I was talking to a peer who was using ChatGPT to help finesse 360 feedback comments about their peers. Because I had invested in the research, I was able to share advice about how to prompt in a way that didn’t inject unintended bias into the comments.
At the time, women and female names were more likely to be associated with passive adjectives- creative, empathetic, etc.- whereas male names were more likely to lead to generation of active words- technical, strong, etc. I advised against using names that would give an indication of gender.
How do you think your experience as a data scientist makes you uniquely suited to educate audiences on gender bias and other biases?
As data scientists, we are tech people who need to see a clear how and why so we can activate. The ability to show numbers and data to back up claims builds credibility.
For less technical users- it’s so important to educate there too. Remediation happens in layers. As data scientists, we help business leaders understand the positioning and application of a model to ensure there is awareness of bias and its implications. Maybe they don't need to see the vector breakdown, but seeing words associated visually in male versus female space context is key.
Visit our knowledge hub
See what you can learn from our latest posts.