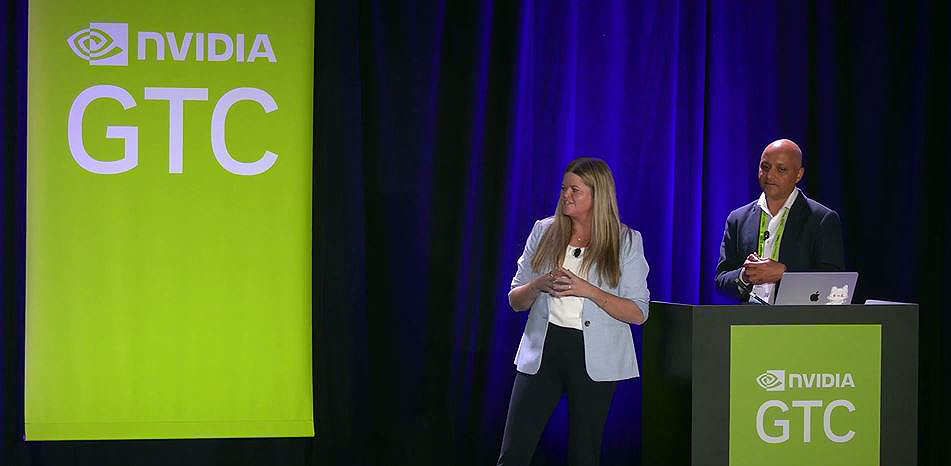
84.51° shares transformative AI journey at Nvidia GTC
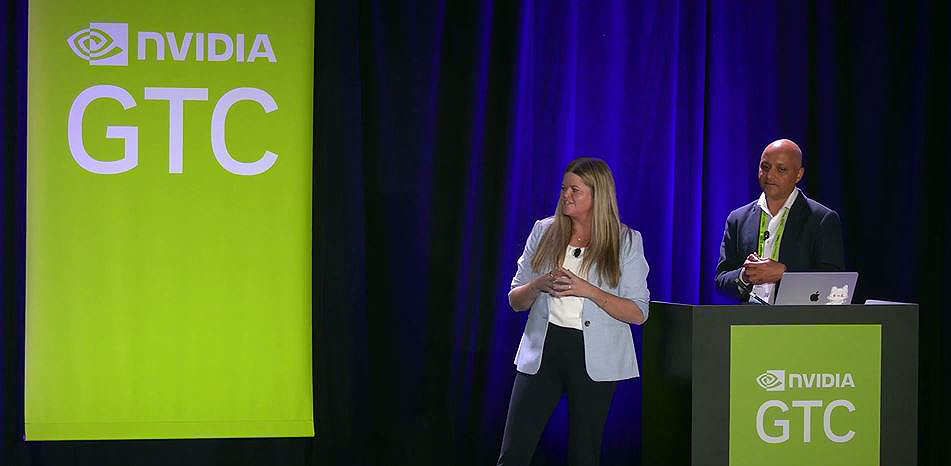
As more organizations recognize the growing ability of artificial intelligence to foster innovation and enhance operations, they’re looking to increase AI implementation to make more informed business decisions. That demand is driving the design and development of the AI factory to enable rapid AI development, improve efficiency, and provide scalable AI decisions across an entire enterprise.
84.51°’s Kristin Foster, senior vice president – AI/Data Science, and Giri Tatavarty, vice-president AI/Data Science, recently discussed the development of Kroger’s AI factory at the Nvidia GTC AI Conference in San Jose, CA. They highlighted their “Hub + Spoke” operating model and how their teams are working to accelerate AI adoption across the enterprise as they research, develop, and deploy customer-first science in grocery retail, insights, customer loyalty, and media. Some of the learnings from their journey include:
Balancing centralized and decentralized AI development
The purpose of the AI Factory is to make AI adoption easier. However, there are barriers for teams to achieve these goals. Barriers to scaling AI can range from difficulty identifying opportunities and proof of concept issues to proof of economics, scale, and continuous improvement. Our mission is to remove barriers at every stage of the funnel and to significantly shorten the time required to progress through it, enabling faster delivery of AI-driven business value.
Our team has many different roles, but one of them is working across a centralized group that ultimately has the mission to make AI better, faster, cheaper, and safer for all of our teams across Kroger – and to make it easier for teams to adopt. That doesn't just come from the science or capabilities we build, it also comes from the changes we need to make to our operating model, our processes, and the frameworks we are giving teams to be able to build these things, as well as the starting points that they all need to be able to build some of these capabilities quickly. We centralized some common AI componentry and brought some AI subject matter experts into a central group.
Speed vs. control in transformative AI
It’s important to establish a balance of speed and control in AI systems. The fast, but messy and fragmented, approach enables rapid experimentation and innovation and allows for quick adaptation to changing market demands. But it results in siloed knowledge and increased duplication, making it less likely to scale. The controlled, but slower, tactic implements governance and standards to ensure quality and compliance, and promotes the reuse of proven methodologies and tools. This strategy supports scalable solutions that can be efficiently and cost-effectively managed, but it can also promote slower innovation that can frustrate teams.
By fostering an environment where innovation and governance co-exist, we can achieve sustainable, scalable progress. We realized that it cannot be done by a technology team or an AI team in a silo or a vacuum, but needs to be done in partnership with the business.
The model matters
The “Hub + Spoke” operating model accelerates value creation, optimizes governance, and maximizes reuse. In a distributed model, each business unit builds and deploys end to end AI on their own, with little to no centralized infrastructure, patterns, or coordination. This may enable speed, but it does not reduce risk or duplication. In a centralized model, all AI is built and deployed by a centralized group, which results in lost domain expertise and a bottleneck of talent. But in the Hub + Spoke model, AI is built and deployed by the business “spokes,” while reusable components, governance, and infrastructure is housed in the “hub.” This accelerates use cases and reduces risk via governance, creating more value via the combination of domain expertise and AI.
The Hub + Spoke model also drives speed to implementation. Business team “spokes” use base capabilities from the hub to focus their efforts on driving topline impact, rather than the “below the line” tasks required to set up and manage Generative AI models and services. We need each team to be able to innovate in their core areas, because they're the ones most intimately familiar with the customer or associate problems we need to solve. Not every team is going to need every capability, but they have the hub that they can go to, and they can pick and choose the things that are going to be most meaningful for their use case. The result is reduced development timelines and fewer resources required.
Evolving the architecture
Over the AI/ML journey so far, our technology stack has evolved in tandem with the broader data and analytics landscape. Today, we're in the age of generative AI, deep learning and agentic patterns, and the underpinning that is powering us is the AI factory. Several technologies power our AI factory, from utilizing Technology & LLM providers like Microsoft and Google for access to frontier models and LLMS. We also embrace Nvidia stack (Triton, Merlin) for creating our own foundation models and serving them.
People ask why you need an AI factory these days when you can spin up LLM in a couple of minutes with any cloud provider. You could do that, but in an enterprise with tens and hundreds of teams, if every team spun up a GPU cluster and used their own tools to create an LLM and endpoint, you’d get a siloed, disconnected architecture – you wouldn’t have enterprise observability, governance, visibility, or the same safety standards across all those LLMs.
What helped us was taking a step back and investing in strategic technologies that are open source and also cloud agnostic, such as Nvidia stack. We also focused on data virtualization, because you can build the greatest AI compute, but if you can't provide it data, it's no good. So we spent time creating that data fabric that connects all these things. And then, finally, we built our AI factory to empower all this. So now we’ve transformed from a siloed, disconnected, vendor-driven architecture to a more unified, scalable, business-driven AI/ML target architecture.
AI Factory outcomes
In its first year, the 84.51°/Kroger AI Factory has produced many tangible benefits. We have a single front door for AI use cases and responsible AI governance. The amount of time required to onboard a new use case has dropped from weeks to less than a day. Our teams have access to foundation models including proprietary models with a single API call, and reusable patterns and reference implementations for common Gen Ai use cases are saving developers hours. It’s been a great journey so far, and we’re looking forward to more great things this year.
For a deep dive on the AI Factory's technical foundations and examples of its impact on use case value, watch the full session. Nvidia has also included a summary of 84.51°’s GTC session in a new eBook featuring successful AI Factory implementations
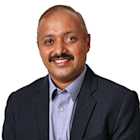
Visit our knowledge hub
See what you can learn from our latest posts.