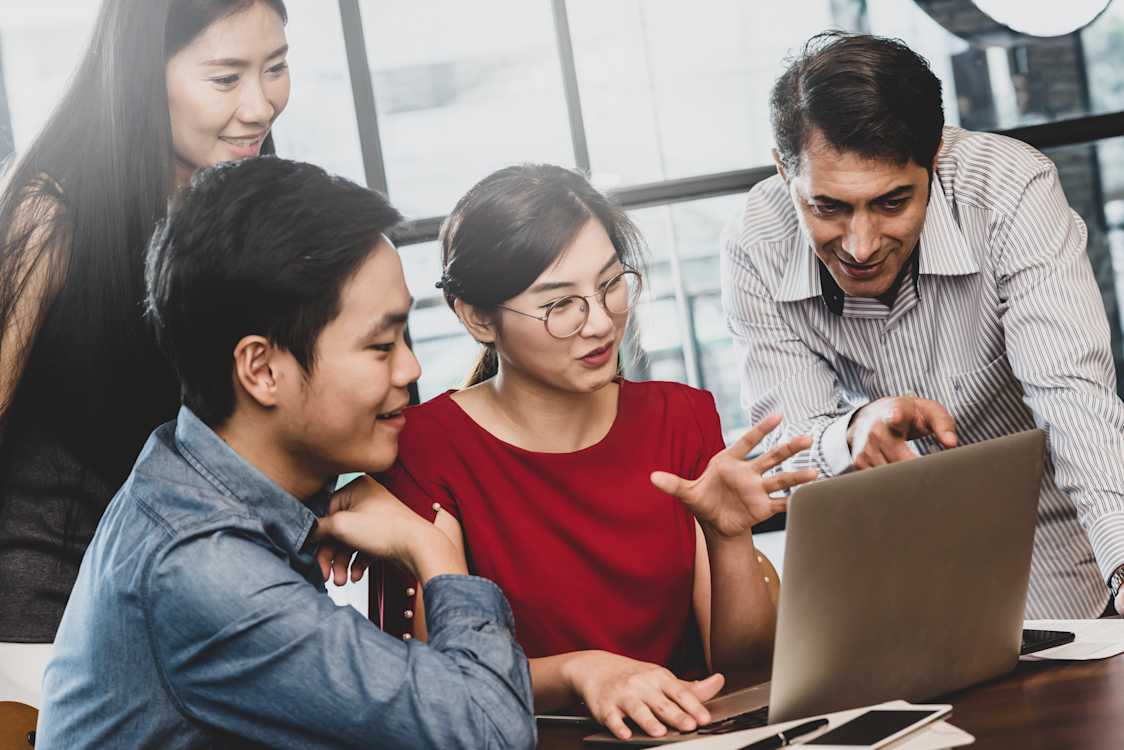
84.51° Data science and retail analytics in action
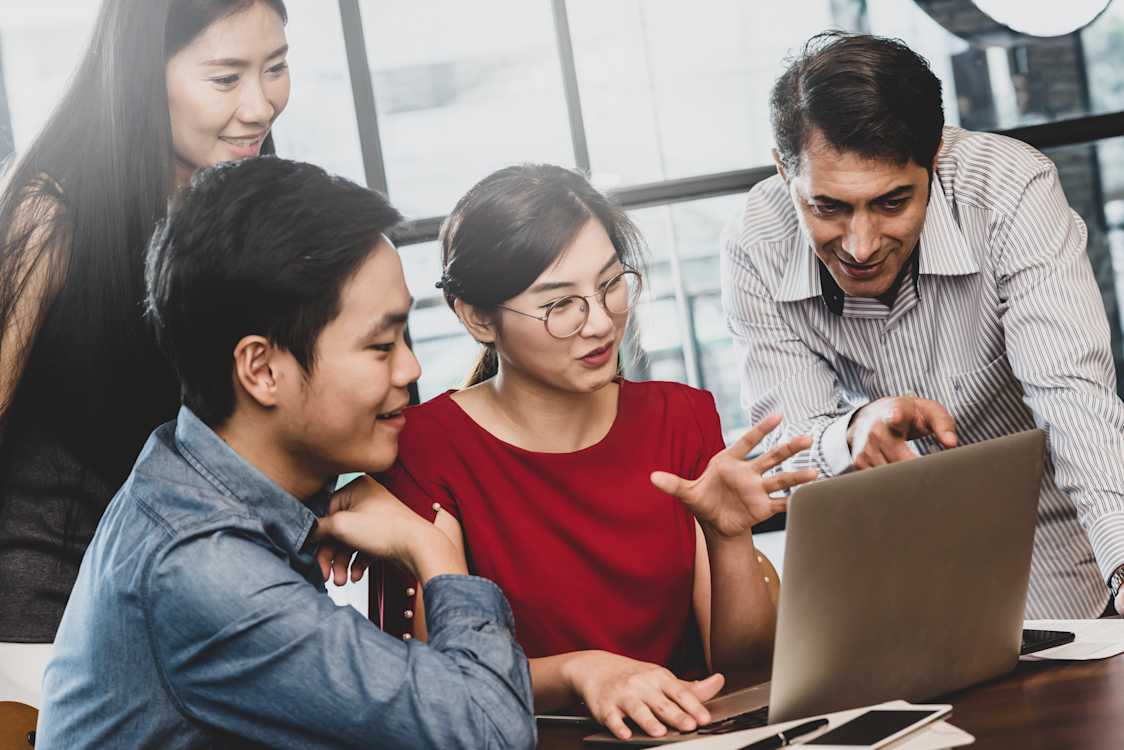
At 84.51°, it’s not just our data that sets us apart – it’s putting what we know about the data into action. By applying our retail data science, insights, and media activation solutions, we help Kroger, brands and other retail businesses develop strategies that help them optimize operations so they can meet their customers where they are with what they need.
Retail analytics power a better shopper experience
Our first-party retail data comes from over 60 million U.S. households and is sourced through the Kroger Plus loyalty card program. Every time a customer swipes their loyalty card at Kroger it does two things: It provides a discount on the items they buy, and it lets us know what they like or dislike from Kroger.
These valuable insights enable us to build meaningful relationships with customers. It allows us to tailor our direct communications to show our customers we see them and understand them. For example, when we analyze the data, we know that it's not efficient to market a honey-roasted ham to someone who’s a vegetarian. We also know that giving someone a $3 coupon, instead of a $2 one, can allow a family to add another item to their basket to make their meal more filling.
Sales data also helps inform retailers on how to optimally run the business, such as how to tailor a store to meet the needs of a community or which products will be most relevant to the community. In addition to helping identify and understand each community’s unique character and preferences, it’s a valuable tool for optimizing the entire retail ecosystem – from streamlining the supply chain and improving inventory management to setting optimal prices.
Data science at work in retail analytics
We apply data science and retail analytics to improve and optimize the shopping experience and influence the entire retail ecosystem. It enables us to (1) look back and extract insights from sources such as customer feedback, financial performance, and operational metrics, and diagnose unexpected behavior (2) develop predictive models such as forecasting and recommender systems (3) prescribe and optimize business decisions based on predictive models and business objectives. It’s a continuously evolving discipline, especially with the advent of artificial intelligence (AI). Researchers have been discussing and experimenting with AI since the 1950s, and the retail industry has been using AI for more than a decade.
Machine learning is a subset of data science that focuses on building models and algorithms that learn patterns and make predictions. It helps people analyze massive amounts of data from multiple sources and find connections between data faster than humans can do. Some of its tools and functions include:
Supervised learning: learns to recognize patterns and relationships between inputs and outputs, allowing it to make predictions on new, unseen data, e.g. forecasting models
Reinforcement learning: machines to learn and make decisions by interacting with their environment, receiving feedback, and optimizing their actions to achieve specific goals, e.g. real time analysis
Optimization: computational procedures used to find the best solution or outcome among a set of possible choices by maximizing or minimizing a specific objective, e.g price optimization
Generative AI: create new content, such as images, text, or music, by learning patterns from existing data, e.g. new product recommendation
Technology advances fueling retail analytics
Our data scientists’ goal is to leverage advancements in technology to build trusted and responsible algorithms to create more valuable experiences for our shoppers. Such algorithms typically involves utilizing multiple tools listed above. We incorporate such advanced algorithms into multiple areas:
Augmented or autonomous processing where we use predictive models (such as forecasting models) and prescriptive sciences (such as optimization) to augment judgement in operations and merchandising. This includes use cases like store ordering, and warehouse optimization.
Intelligent media product offerings we deploy in analytical product offers that are complete with model monitoring and interpretability. We see this come to life through our Kroger Precision Marketing (KPM) platform, where we have optimized audiences that brands can utilize to maximize ROI for their media campaigns.
Robust insights or decision intelligence where our advanced analytics and machine learning is embedded throughout the enterprise to support decisions – such as our customized assortment and shelf optimization sciences.
All around personalization, using AI to create personalized, relevant, unique experiences for our shoppers whether it is the prices they pay, their experience on the Kroger app, the content and creative offers they receive, or the products they see.
Manufacturing we utilize the latest AI agentic framework to enable us to optimize formulations and rapidly speed up development of new and innovative products to satisfy evolving customer taste and preferences
We already have AI and machine learning embedded in many places across our ecosystem that has demonstrated tangible business value. While the buzz around AI is loud, generating value out of such algorithms is hard – something we have successfully demonstrated repeatedly across our business. Across merchandising, for instance, there are optimization and forecasting sciences to drive pricing, promotion and assortment decisions. These advanced machine learning algorithms are fed into systems and processes to create a better customer experience and make the lives of merchants easier. Within the digital e-commerce experience, deep learning algorithms make it easier for customers to save time and money while ensuring they receive the most relevant products and offers for them. And we are finding ways to elevate and amplify the voice of the customer through improved sentiment analysis.
We are also leveraging AI across stores, supply chain (transportation and warehouses), HR, and customer experience. We will continue to invest in new capabilities and create scalable and democratized frameworks for AI, to embed AI in all we do.
For example, Sage is a personalized AI virtual assistant that puts labor data and metrics at the fingertips of store leaders. VROOM is a machine learning model that optimizes outbound truck routing by trying out thousands of possible route options and building the optimal route. IRIS leverages data intelligence to improve sell through rate based on a specified sell through window, such as a season or holiday. And Quevision leverages AI to predict traffic in checkout lanes, allowing for better planning of staffing to reduce customer wait times, creating a better associate and customer experience. We also continue to build out the next generation of optimization sciences to leverage price & promotion together to improve category performance and create a division-level executable sales plan.
Optimizing supply chains with retail analytics
Another example of the power of data science and retail analytics lies in supply chain transportation optimization. In the retail industry, current supply chain movements are siloed, disconnected from the business, and handled manually. Also, applying retail analytics tools can help organizations optimize all supply chain movements to create a future where every transportation decision is connected to the customer, overall business and its environmental impact.
Route optimization is a retail industry challenge, and as size increases, it becomes very difficult to solve this problem fast. Vehicle routing faces a myriad of constraints including time windows of locations, resources available (vehicles and labor), vehicle capacity, types of goods (frozen or perishable items that have a shelf life), and unpredictable traffic and roads.
The typical routing system in place today is a manual process with potential for evolution. A data science-based solution is fully automated and uses cutting-edge science to optimize the entire fleet. Because retailers spend significant amounts of money on outbound transportation annually, every percentage of improvement in truck routing results in cost savings and reduced environmental impact. This solution also offers ethical benefits by contributing to a more sustainable supply chain.
We have demonstrated that such solutions when scaled across the retail business have the potential to significantly enhance transportation and adds significant business value. For example, for the US transportation industry, which is more than $40B in size and contributes to over 300 billion miles every year, a mere 5% saving through optimization presents a value proposition of $2B in saving and 15B less miles.
Learn more about retail analytics in action
Learn more about how data science and retail analytics deliver performance for retailers and brands – and value for shoppers – through our Insights and Research & Analytics solutions.
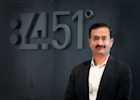
Visit our knowledge hub
See what you can learn from our latest posts.