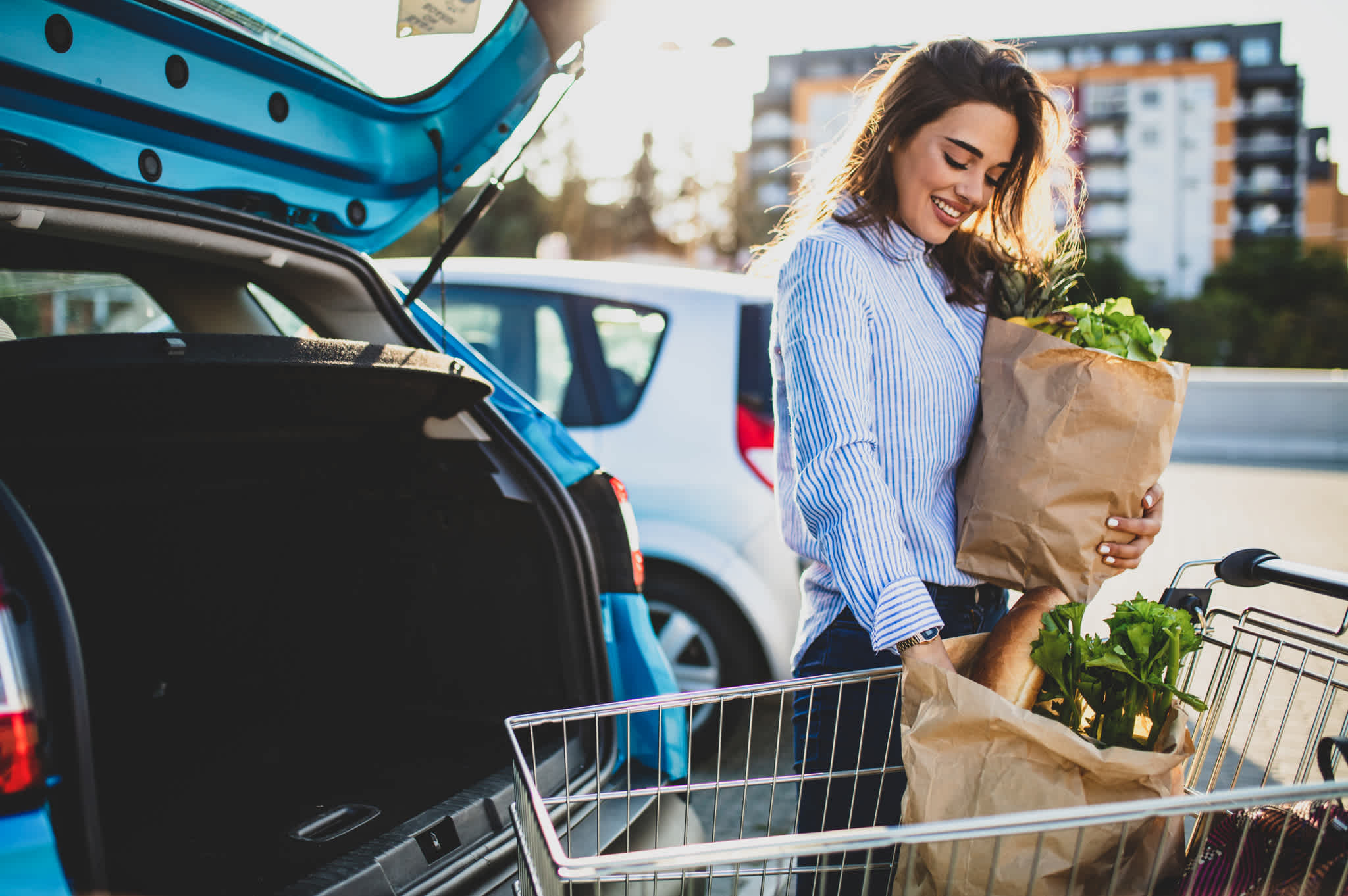
Optimizing omnichannel fulfillment with machine learning
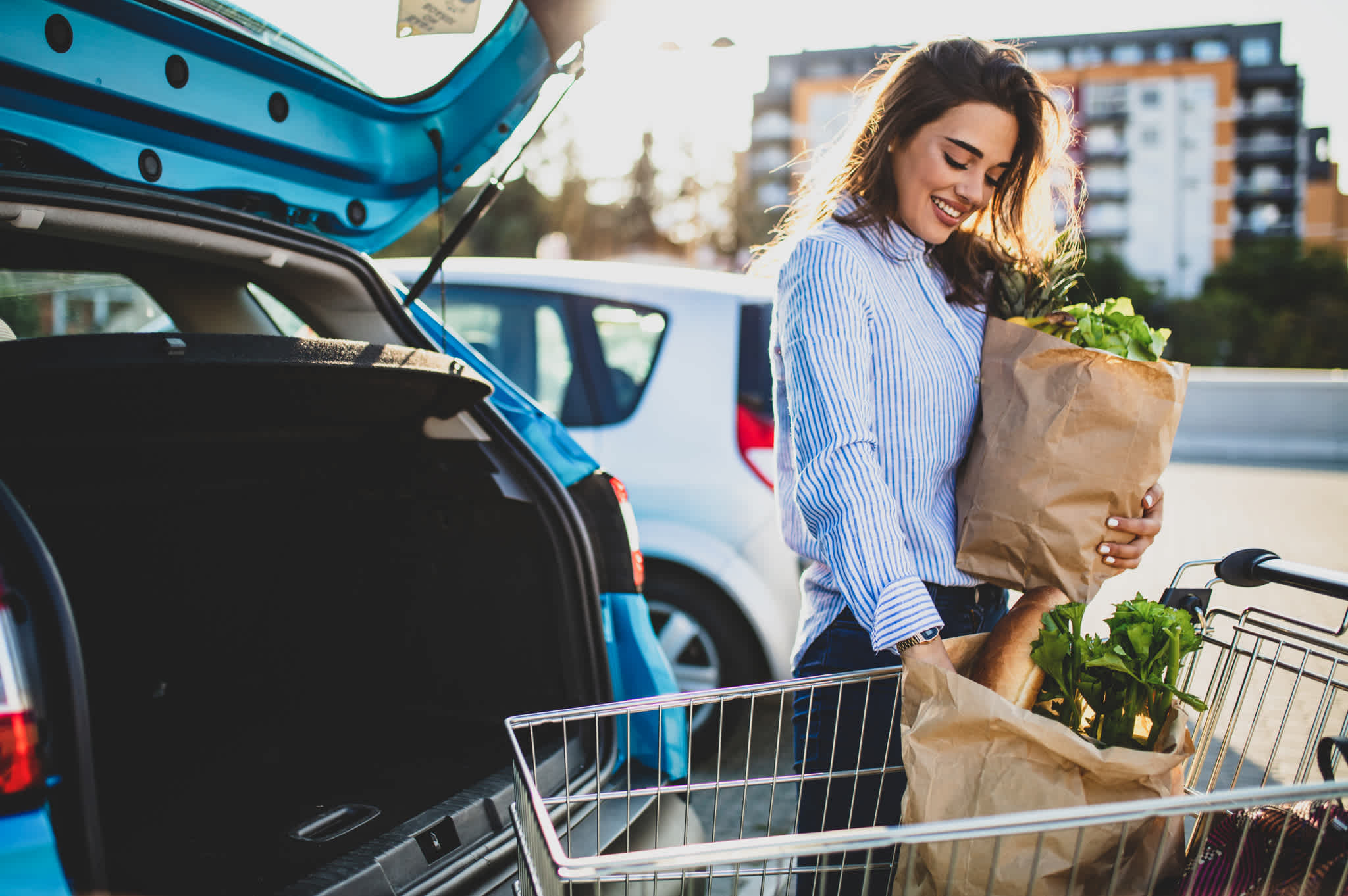
Hybrid shopping is here to stay. As the number of online shoppers continues to rise post-COVID, so do customer expectations for seamless omnichannel experiences. Whether they choose curbside pickup or delivery service, these customers want increasingly fast and flexible options.
Those demands are prompting retailers to take a closer look at how their omnichannel fulfillment services can best complete those orders. With the demand for fulfillment services rapidly increasing, innovative solutions like dynamic batching and other tactics are needed to help consumer packaged goods (CPG) companies and retailers keep up with customer demand.
Smarter trolley travel
In-store fulfillment operations typically use wheeled trolleys that hold different numbers of totes. The store pickers move these trolleys around the floor while placing specified order items into the appropriate tote. The question is, how do we decide which totes belong together to be filled at the same time? Currently, many retailers make those decisions based on the time the bags are due.
Dynamic batching, also known as smart batching, adds more variables to that equation — the most important of which is the route that the trolley takes through the store in order to fill it.
One type of batching might group similar items: If you had orders with lots of produce in them, it might make sense to make a trolley consisting of totes that are produce-heavy, so that the trolley doesn’t have to move around the floor as much.
While it seems like it would be simple to group items based on product category, the reality is that product categories are often spread out across the store. So we're developing technology that uses machine learning to help pickers work more efficiently. In one instance, they might group produce with something frozen nearby; the next time they might group produce with something else in a different aisle nearby. Every order is different and the combinations change every day.
Better batching, faster results
Other strategic processes can work in tandem with dynamic batching to optimize fulfillment. A process called cubing applies the same logic to dividing orders into similar bags. The other process is routing, where we tell the pickers in what order to pick up items in the store to minimize the distance to travel.
We’re looking at reprogramming the handheld devices that direct the pickers to minimize the distance traveled. Preliminary testing on this process indicates a strong improvement in the efficiency over the way these devices currently route pickers through the stores.
Optimizing the customer experience
There are two aspects of dynamic batching that will also positively impact customers and CPGs. The first is that it will enable retailers to lower their fulfillment lead time, or the minimum amount of time required between placing an order and receiving it. Testing shows reducing fulfillment lead time leads to an increase in orders and sales as well as households engaging in pickup services. With shorter lead times, customers are better able to place an order on the way home from work or when realizing they forgot an ingredient for something, which boosts orders.
The second impact is an increase in customer capacity. Currently, there’s a cap on the number of orders that can be placed in a certain time window. We’ve found that during the rush hours right after work, those time slot windows fill up immediately. But if fulfillment services can be more efficient with the same amount of labor, then we can take more orders in the same time window. Allowing customers to place more orders in the same timeframe also results in happy customers who are more likely to become loyal customers. This is just one example of how incremental changes such as improving in-store fulfillment operations can have an outsize impact on the customer experience.
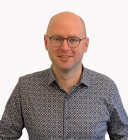
Visit our knowledge hub
See what you can learn from our latest posts.