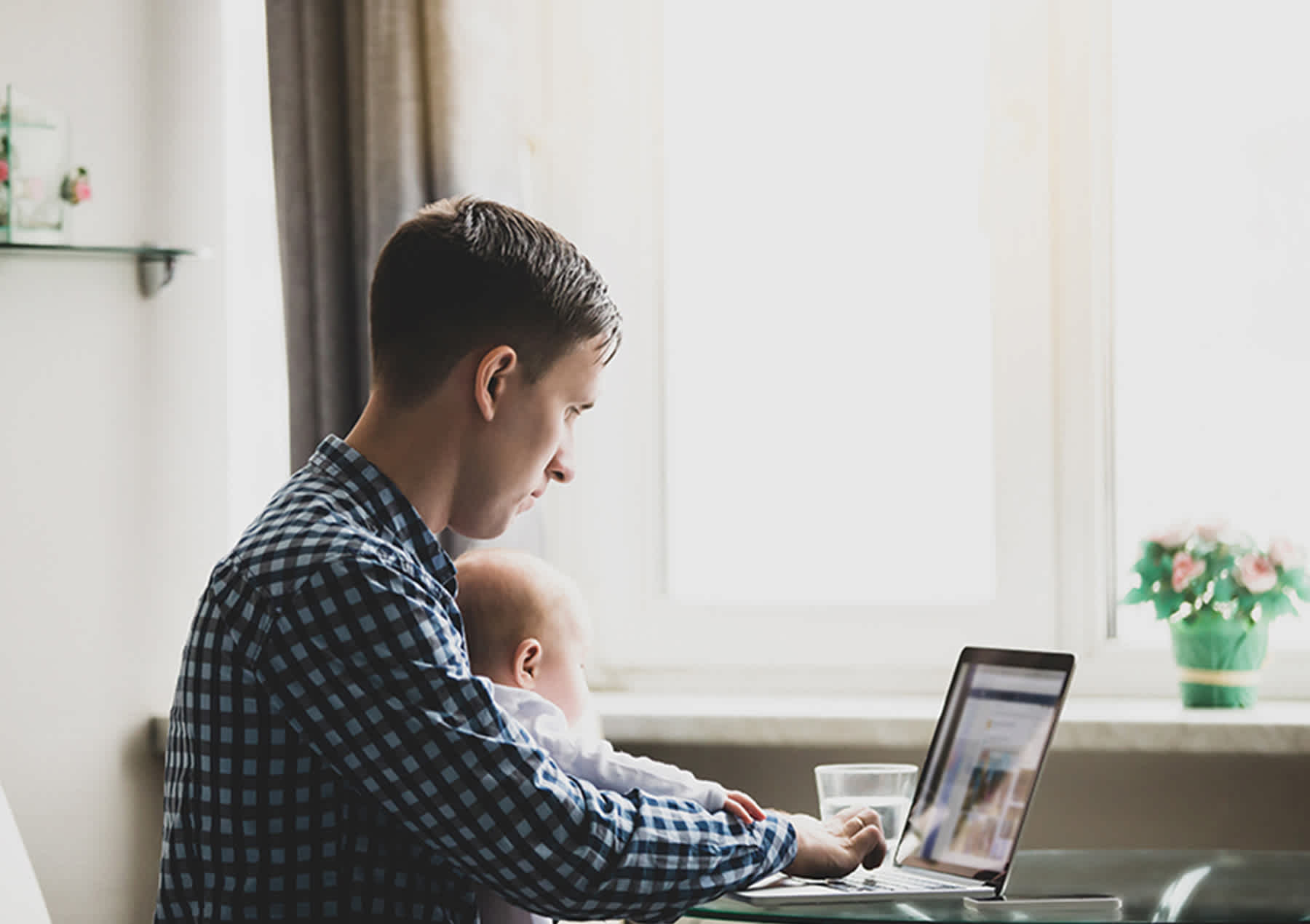
Why data science is important for organizations today
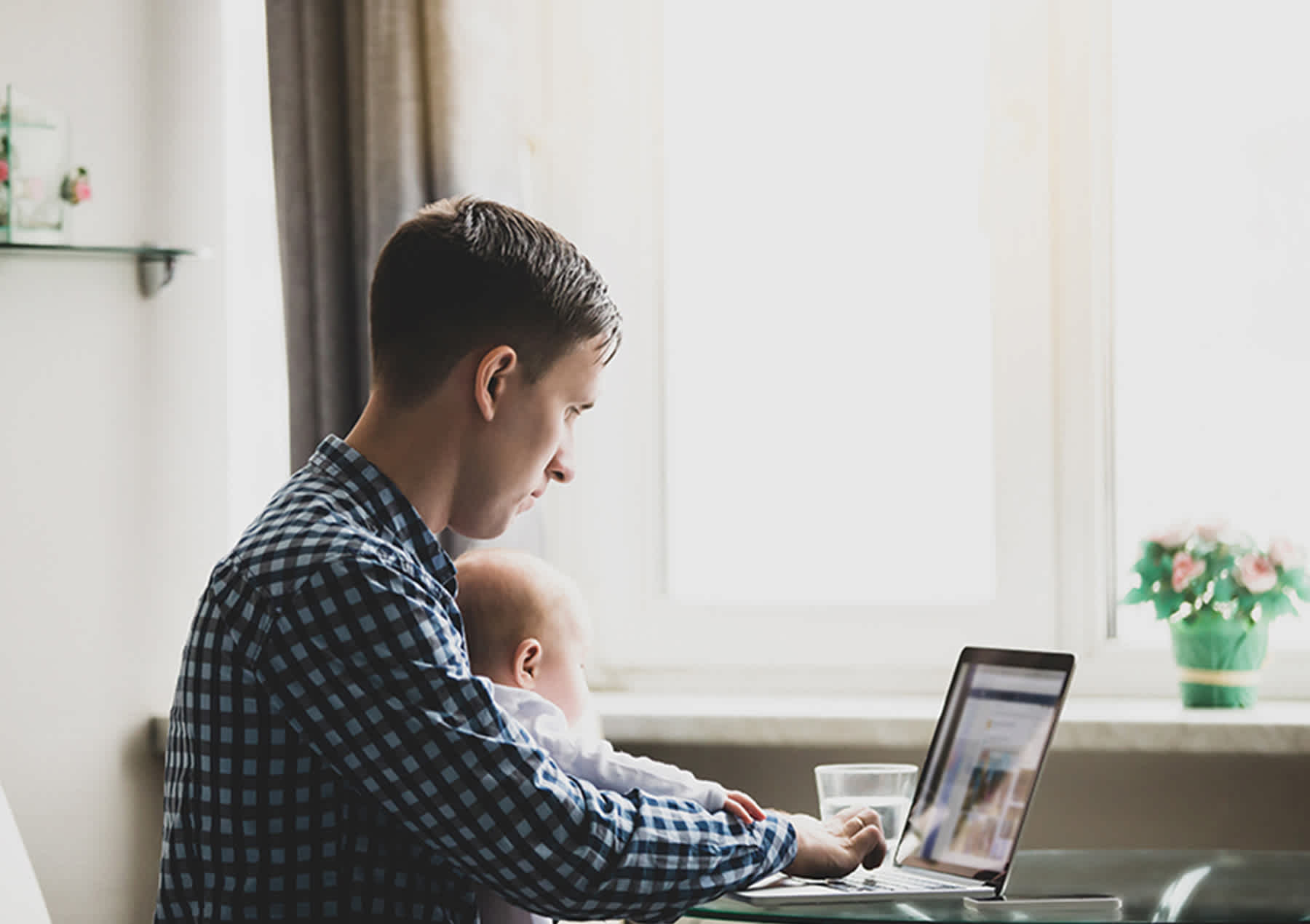
Since becoming known as the “sexiest job of the 21st century” in 2012, small data science startups have popped up everywhere. Often times, these small groups are touting that they have developed “game-changing algorithms,” or have access to novel data that will substantially improve an organization’s outcomes. While many of these small companies have demonstrated the ability to provide great benefit, others have caused an extensive amount of turbulence in industries such as digital retail and grocery. As organizations continuously try to cull more value from their data, many such startups are making blind promises in an effort to drive revenue.
Significant time and effort have gone into the development of sciences or analyzing data to help drive operational outcomes or business value. Motivation often derives from the development of material that positively impacts people’s lives. Whether this comes in the form of building a model that brings consumers closer to products they want, or helping to identify medical ailments more effectively, data scientists tackle hard problems that make a difference in industries ranging from consumer packaged goods and retail to healthcare and media. Other sectors that rely heavily on data science to understand markets and predict industry and customer activity include finance, manufacturing, transportation and energy.
Today, data science can actually help an organization gain credibility. While there is plenty of discourse around gaining credibility as an individual, I wanted to expand this discussion to the case where an entire team, function, or capability is attempting to gain credibility. To consider gaining credibility, it is important to have a good understanding of what credibility means. Here, our focus is on being the team trusted with delivering the data science needs for your organization where deliverables are actionable, and not shelved for the sake of intuition.
Paramount to establishing any type of credibility are three main characteristics: quality, consistency, and trust. For most, these are no-brainers, but the challenge we face as leaders is persistent organizational resistance while establishing these as core values within our team. While there may be other characteristics that directly impact credibility, such as integrity and ethical use of data, many of them are encapsulated in the three laid out below.
The definition of quality provides a great foundation for why it drives credibility: "The standard of something as measured against other things of a similar kind; the degree of excellence of something."
Because of the potential impact it has on decision making and driving value into the business, quality is a primary foundation on which credibility is established. Here, quality includes other features such as morality, truthfulness, and value, amongst others. Whether it’s a one-off analysis, a perpetually used science, presentation of results, or a white paper, credibility is established through excellence of the product. Excellence could mean many things. For 84.51°, it means helping improve our customers’ lives, allowing our executives to make more informed decisions, identifying new business opportunities, automating critical business functions, and so much more.
When complete, an analysis should answer the business question in a concise, yet clear manner – clearly enumerating the assumptions, discussing robustness of the results, and providing the necessary information needed for the outcome of the effort. As will be discussed in a later section, many leaders aren’t aware of what they don’t know, so a quality analysis provides them access to this level of information in a succinct fashion.
Subject matter expertise can go a long way in ensuring quality, but as is discussed later, it’s not the only piece. An irrefutable product becomes a starting point for ensuring a team’s quality proposition. Quality should also improve as time goes on through research, feedback, and ongoing education. Depending on the level of maturity of the data science organization, and the ability for the enterprise to adopt this mindset, quality may vary. By ultimately demonstrating industry leadership in data science and the relevant industrial domain, quality will continue to improve, and can spread virally throughout the organization.
Imagine a senior leader asking a question to three people, all who come back with different answers. Because of our individuality, this hyperbole is actually quite abundant in industry analysis, and very quickly degrades credibility. This pervasiveness compounds itself in an organization that has multiple, independent teams delivering data science for a large organization or a wide variety of clients. Just as low-variability processes improve planning, consistency in delivering quality results encourages management to keep coming back for more. A consistent message across all stakeholders provides a paramount pillar to building credibility. To help foster an environment of consistency, there are various initiatives a data science team can look to:
Numerous benefits can come from embedding consistent practices across your data science organization. In addition to simplifying onboarding and training of new employees, standardization supports interpretability and ensures analyses are repeatable and answer business questions in a consistent manner.
First, and foremost, should be an effort to develop standardized methods for data use, or what 84.51° calls Golden Rules, within the company. These rules form the basis of defining what data should be used for certain types of analysis. Golden rules become especially important as the volume of data increases and are stored in various locations within a data lake. In general, this helps to ensure all data scientists are using similar data throughout development.
Next, development of a machine learning pipeline can provide a modular-like approach to developing and productionizing algorithms. By taking the time to engineer processes that data scientists can plug into, an organization can make huge strides toward ensuring consistent delivery of high-quality decision systems.
Depending on how science comes to life within an organization, unified application programming interfaces (APIs) provide a huge benefit to ingesting your developed sciences. Instead of having a single API for each individual tool, consider aggregating them at a business unit level, making sure your consumers have a consistent means for accessing your services.
Automation can be one of the most powerful tools in ensuring consistency within a data science organization. At 84.51°, automation is a force multiplier that frees up our valuable assets, and is a foundational mindset.
Automated analysis tools have been around a while and can also accelerate an organization’s ability to rapidly deploy machine learning models in a consistent fashion. Use of an organization’s Golden Rules, coupled with automated analysis tools provides a means for quickly identifying winning algorithms to help solve the business’s most pressing problems.
Something else often found within organizations that is ripe for automation is development of analyses reporting business outcomes. Reports can come in many flavors – briefings, dashboards, automated emails – but they’re all designed to notify the recipient of the status of some metric of interest. As is common with engineering practices, data scientists should incorporate automatic notification when it appears their algorithms are outputting anomalous behavior. Additionally, for business leaders, while reports can often start off as ad-hoc requests, they often turn into ongoing needs. Data scientists should pay special attention to these seemingly one-off requests and automate anything that has the potential to be repeated.
Finally, as tools, algorithms, and services should all be monitored and measured for quality, bias and drift, development of an automated measurement platform can help drive consistent outcomes. Automated measurement can cover anything from basic feedback of client campaign analysis to A/B or multi-armed bandit test and evaluation on an eCommerce platform. Several such tools exist as commercial off the shelf (COTS) products, so an organization should consider whether or not procurement makes sense, or if it is worth the time investing in development of their own. In general, as the size of the organization, volume of data, or complexity of sciences increases, investment in internal tools makes more sense.
Through standardized processes and automation, a data science organization can quickly demonstrate the consistency necessary for establishing credibility amongst their consumers.
The final pillar in establishing credibility is trust, which is generally a direct biproduct of quality and consistency. There are, however, other aspects that help to build trust. One such aspect is the importance of knowing and articulating your limitations. The organization must have a strategic perspective on data science capabilities they want in-house and a cost-effective plan for sourcing the remaining from external partners. Many such technologies have been comprehensively addressed by tech companies, but to begin developing this data vision, there must be a baseline level of scientific savvy.
As senior leaders continue jumping on the data train, few of them are fully exploiting the potential it can have on their business’s success. Establishing credibility can be one of the most critical components to accelerating these capabilities of your data science organization. While some ownership is in the hands of these key stakeholders, it is ultimately the job of the data scientists to provide quality analyses in a consistent fashion so that trust is established. And at the foundation of credibility is trust.
Visit our knowledge hub
See what you can learn from our latest posts.