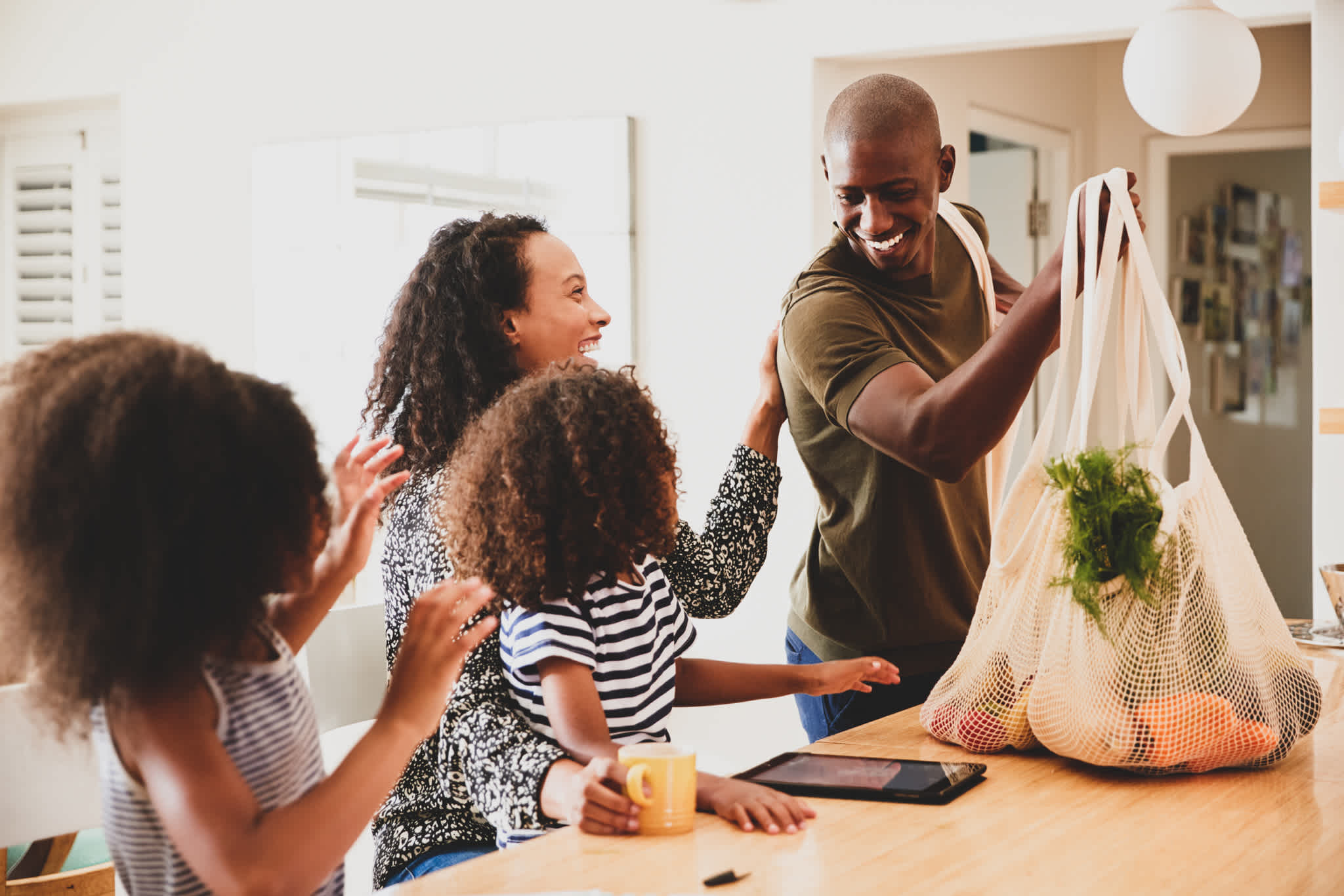
How to crack the code on top-selling product pairings
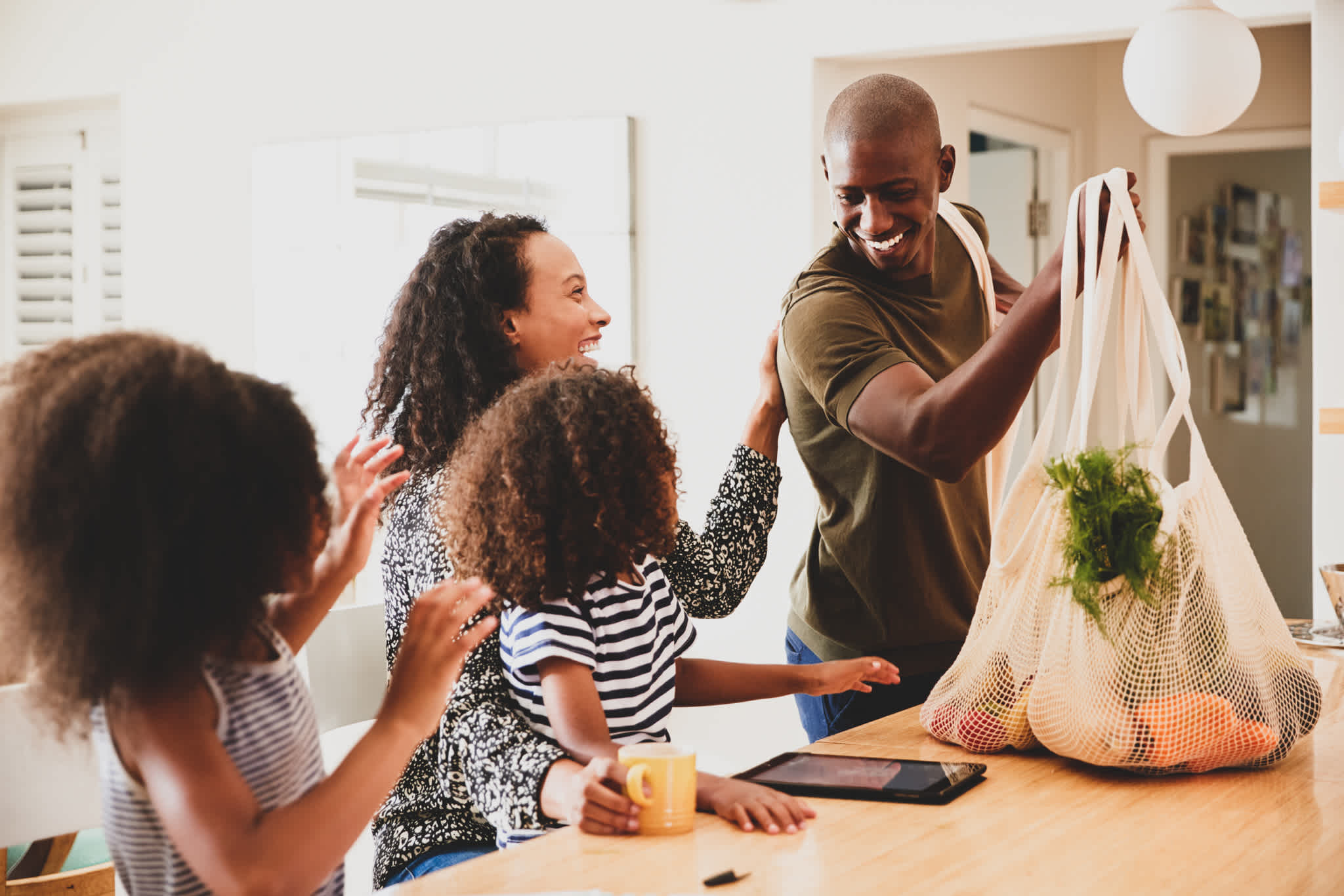
There is an art and a science to understanding which products are best combined in a promotion, on shelves and other pairings. A cross-shop analysis provides a compass to navigating these opportunities. We’ll explore three essential cross-shop techniques that serve different business objectives and how to utilize them effectively.
What is a cross-shop analysis?
Cross-shop analysis involves examining the purchasing behavior of shoppers to identify patterns and relationships between different products. By understanding which products are frequently bought together or substituted for one another, brands can gain insights into consumer preferences and make data-driven decisions to improve their offerings.
Cross-shop analysis answers questions such as:
How can I identify popular product combinations?
Is there a meaningful cross-shop relationship between my two product groups during a single trip?
What are the overlaps and interactions between products and brands?
What competitive products are my customers purchasing over time?
Types of cross-shop analysis
There are three key cross-shop techniques, each valuable for different purposes:
1. Basket pairings: Basket level cross-shop analysis looks at items purchased together during a given shopping trip. This type of analysis is useful for identifying complementary product pairings and potential adjacency opportunities between products. For example, if peanut butter and jelly are frequently bought together, placing these items near each other could drive more sales.
Use cases:
Identifying popular product combinations
Finding potential adjacency opportunities
Identifying differentiation or overlap between products
2. Supplemental pairings: This analysis calculates the percentage of baskets that contain both products A and B. This helps brands understand how frequently two product groups are both purchased and identify opportunities to promote both products together. For instance, an analysis of cookie dough and white milk sales at Kroger stores revealed that two weeks before Christmas, 89% of baskets contained only white milk; 7% of baskets contained both and 4% only contained cookie dough (source: 84.51° Stratum Cross Shop: 2 Way Venn Diagram).
In addition to analyzing the percentage of baskets that contain both products A and B, it is valuable to examine the number of baskets that contain only one of the products. For instance, understanding how many baskets contain only white milk or only cookie dough provides insights into the scale of one-way interactions. This information is crucial because if two items are often bought together but only by a few households, the potential impact of promoting them as a pair is limited. By identifying these one-way interactions, brands can better assess the true scale of product pairings and make more informed decisions about which products to promote together.
Use cases:
Understanding overlaps and interactions between products and brands
Identifying products, sizes or flavors to promote together
Identify the size of prize (or scale) of a product pairing
3. Household level analysis Household level cross-shop analysis examines items purchased over time by a household. This type of analysis is useful for determining how long households take to repurchase certain products, identifying competitive products and gaining new households. With this analysis, for example, a brand could look for households that have only purchased from high-indexing competitive products and send a coupon to try their product instead. Use cases:
Identifying competitive product sets
Finding product pairings purchased in succession
Exploring potential adjacency opportunities
Pro tip: Cross-shop analysis can be time-consuming to build out due to the complexity of data collection, integration and interpretation. Sifting through vast amounts of data to identify meaningful patterns and insights is a daunting task without the right tools. This is why a solution like 84.51° Collaborative Cloud is invaluable. It comes with built-in analytical capabilities that streamline the cross-shop analysis, allowing brands to save time and resources. Read our ebook, “Navigate the data jungle: The quest to find the missing sales” to learn about other key data science techniques and tools.
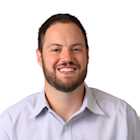
Visit our knowledge hub
See what you can learn from our latest posts.