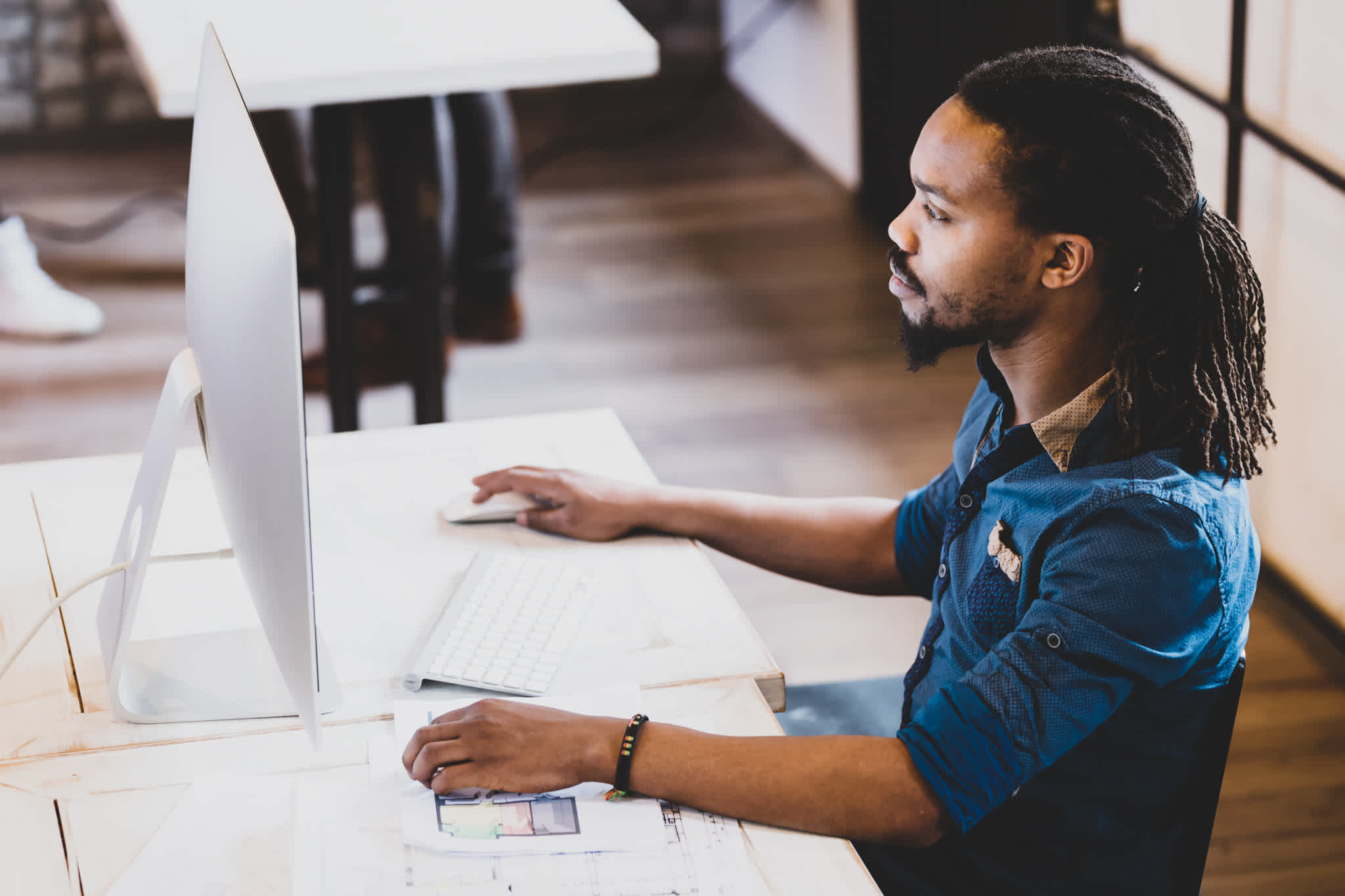
How data science can help an organization gain credibility
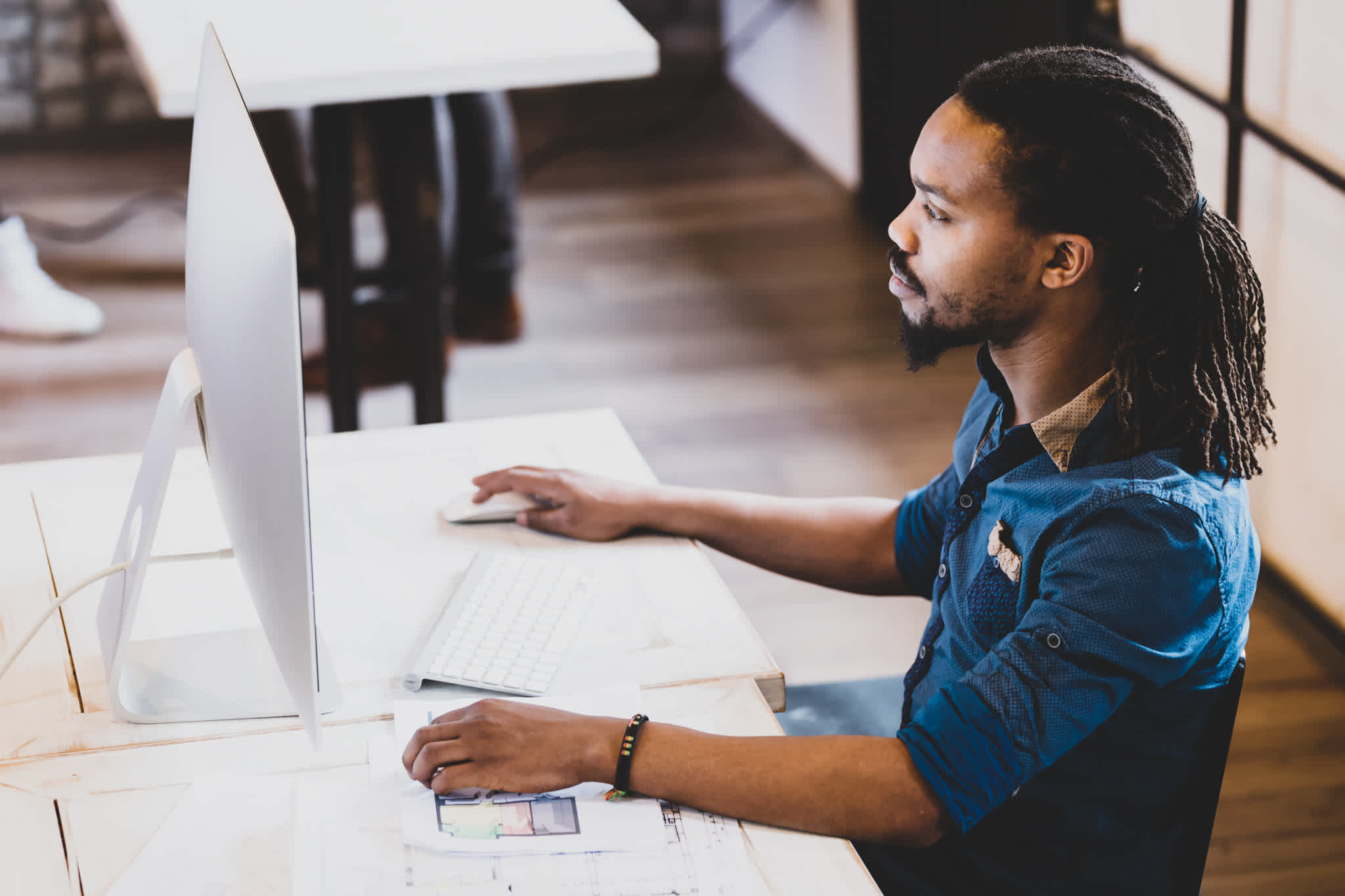
Introduction
After recently listening to a podcast, I started thinking about my past experiences providing data analysis for several large organizations in my career. Many times, significant time and effort has gone into the development of sciences or analyzing data to help drive operational outcomes or business value, yet little action occurs as an outcome. If you’re anything like me, motivation derives from the development of material that positively impacts people’s lives. Whether this comes in the form of building a model that brings consumers closer to products they want, or helping to identify medical ailments more effectively, data scientists tackle hard problems that make a difference. What is interesting is that given the ubiquity of examples where data science has driven value for organizations, hard work still gets shelved and key business decisions are made on “gut” or “experience.” This can be especially true in fragmented organizations where data science is a standalone capability not fully integrated within the fabric of the company culture. In these cases, the challenge lies in becoming the trusted agents that leaders go to – a challenge of credibility.
Since becoming known as the “sexiest job of the 21st century” in 2012, small data science startups have popped up everywhere. Often times, these small groups are touting that they have developed “game-changing algorithms,” or have access to novel data that will substantially improve an organization’s outcomes. While many of these small companies have demonstrated the ability to provide great benefit, others have caused an extensive amount of turbulence in industries such as digital retail and grocery. As organizations continuously try to cull more value from their data, many such startups are making blind promises in an effort to drive revenue. While there is plenty of discourse around gaining credibility as an individual, I wanted to expand this discussion to the case where an entire team, function, or capability is attempting to gain credibility. To consider gaining credibility, it is important to have a good understanding of what credibility means. Here, our focus is on being the team trusted with delivering the data science needs for your organization where deliverables are actionable, and not shelved for the sake of intuition.
Three principal characteristics of credibility
Paramount to establishing any type of credibility are three main characteristics: quality, consistency, and trust. For most, these are no-brainers, but the challenge we face as leaders is persistent organizational resistance while establishing these as core values within our team. While there may be other characteristics that directly impact credibility, such as integrity and ethical use of data, many of them are encapsulated in the three laid out below.
Quality
In writing this, I took the time to look up the specific definitions for each of our characteristics which can help lead to credibility. The definition of quality provides a great foundation for why it drives credibility:
"the standard of something as measured against other things of a similar kind; the degree of excellence of something."
Because of the potential impact it has on decision making and driving value into the business, quality is a primary foundation on which credibility is established. Here, quality includes other features such as morality, truthfulness, and value, amongst others. Whether it’s a one-off analysis, a perpetually used science, presentation of results, or a white paper, credibility is established through excellence of the product. Excellence could mean many things. For 84.51°, it means helping improve our customers’ lives, allowing our executives to make more informed decisions, identifying new business opportunities, automating critical business functions, and so much more.
When complete, an analysis should answer the business question in a concise, yet clear manner – clearly enumerating the assumptions, discussing robustness of the results, and providing the necessary information needed for the outcome of the effort. As will be discussed in a later section, many leaders aren’t aware of what they don’t know, so a quality analysis provides them access to this level of information in a succinct fashion.
Subject matter expertise can go a long way in ensuring quality, but as is discussed later, it’s not the only piece. An irrefutable product becomes a starting point for ensuring a team’s quality proposition. Quality should also improve as time goes on through research, feedback, and ongoing education. Depending on the level of maturity of the data science organization, and the ability for the enterprise to adopt this mindset, quality may vary. By ultimately demonstrating industry leadership in data science and the relevant industrial domain, quality will continue to improve, and can spread virally throughout the organization.
Consistency
Imagine a senior leader asking a question to three people, all who come back with different answers. Because of our individuality, this hyperbole is actually quite abundant in industry analysis, and very quickly degrades credibility. This pervasiveness compounds itself in an organization that has multiple, independent teams delivering data science for a large organization or a wide variety of clients. Just as low-variability processes improve planning, consistency in delivering quality results encourages management to keep coming back for more. A consistent message across all stakeholders provides a paramount pillar to building credibility. To help foster an environment of consistency, there are various initiatives a data science team can look to:
Standardized processes
Numerous benefits can come from embedding consistent practices across your data science organization. In addition to simplifying onboarding and training of new employees, standardization supports interpretability and ensures analyses are repeatable and answer business questions in a consistent manner.
First, and foremost, should be an effort to develop standardized methods for data use, or what 84.51° calls Golden Rules, within the company. These rules form the basis of defining what data should be used for certain types of analysis. Golden rules become especially important as the volume of data increases and are stored in various locations within a data lake. While there are some occasions that will slip through the cracks, in general, this helps to ensure all data scientists are using similar data throughout development.
Next, development of a machine learning pipeline can provide a modular-like approach to developing and productionizing algorithms. By taking the time to engineer processes that data scientists can plug into, an organization can make huge strides toward ensuring consistent delivery of high-quality decision systems.
Depending on how science comes to life within an organization, unified application programming interfaces (APIs) provide a huge benefit to ingesting your developed sciences. Instead of having a single API for each individual tool, consider aggregating them at a business unit level, making sure your consumers have a consistent means for accessing your services.
Automated tasks
Automation can be one of the most powerful tools in ensuring consistency within a data science organization. At 84.51°, automation is a force multiplier that frees up our valuable assets, and is a foundational mindset.
Automated analysis tools have been around a while and can also accelerate an organization’s ability to rapidly deploy machine learning models in a consistent fashion. Use of an organization’s Golden Rules, coupled with automated analysis tools provides a means for quickly identifying winning algorithms to help solve the business’s most pressing problems.
Something else often found within organizations that is ripe for automation is development of analyses reporting business outcomes. Reports can come in many flavors – briefings, dashboards, automated emails – but they’re all designed to notify the recipient of the status of some metric of interest. As is common with engineering practices, data scientists should incorporate automatic notification when it appears their algorithms are outputting anomalous behavior. Additionally, for business leaders, while reports can often start off as ad-hoc requests, they often turn into ongoing needs. Data scientists should pay special attention to these seemingly one-off requests and automate anything that has the potential to be repeated.
Finally, as tools, algorithms, and services should all be monitored and measured for quality, development of an automated measurement platform can help drive consistent outcomes. Automated measurement can cover anything from basic feedback of client campaign analysis to A/B or multi-armed bandit test and evaluation on an eCommerce platform. Several such tools exist as commercial off the shelf (COTS) products, so an organization should consider whether or not procurement makes sense, or if it is worth the time investing in development of their own. In general, as the size of the organization, volume of data, or complexity of sciences increases, investment in internal tools makes more sense.
Through standardized processes and automation, a data science organization can quickly demonstrate the consistency necessary for establishing credibility amongst their consumers.
Trust
The final pillar in establishing credibility is trust, which is generally a direct biproduct of quality and consistency. There are, however, other aspects that help to build trust. One such aspect is the importance of knowing and articulating your limitations. The organization must have a strategic perspective on data science capabilities they want in-house and a cost-effective plan for sourcing the remaining from external partners. Many such technologies have been comprehensively addressed by tech companies, but to begin developing this data vision, there must be a baseline level of scientific savvy.
Organizational Literacy
One aspect that generally lacks in sufficient dialogue is that credibility is not simply something to be gained by one side of the house. Rather, it should be an agreement between two parties. Data science has moved far beyond business intelligence, and it’s time for companies to move beyond simply digesting what has happened and start shaping what* will happen* by educating themselves in the mystic arts of data and science.
In addition to continually upskilling our data scientists, we emphasize scientific thinking throughout the entirety of our enterprise. To do so, 84.51° has established an enterprise-wide data science academy to ensure we continually maximize our organizational data science IQ. This educational capability combines internally developed courses, externally sourced material and a consultative program that aims to directly apply learnings to a relevant business problem. These robust curricula are intended to emphasize a much more comprehensive learning environment beyond what is gained from traditional classroom or e-learning environments.
Literacy also goes both ways. As the more operationally focused members should upskill in the ways of science, so should the scientists upskill in the needs of the business. Credibility improves as this type of collaborative discourse increases, because each member of the team becomes able to understand what each other is thinking about and can clearly articulate how data and science are directly impacting business outcomes. As literacy increases, the credibility gained through quality and consistency should only expand.
Communication
Once a baseline of organizational literacy has been established, communication becomes crucial for establishing trust. It becomes the responsibility of the data science organization to provide the right amount of information to the right people at the right time. In many cases, leadership looks for ad-hoc insights to help build a story or make a critical decision. Further, as our methodologies continue to advance, data scientists face the ever more pressing issue of interpretability of our analyses.
As relationships are established between the data science community and key stakeholders, here are a list of things to communicate:
Capabilities – your customers need to have a baseline understanding of your scope. Are you here to provide them with regular updates of how the business is performing or are you coming with prescriptive analyses to inform strategy? Clearly identifying what’s in your wheelhouse avoids significant confusion as the relationship continues to build.
Process – describe what they can expect from your team. During this communication, things such as the overall evaluation criteria (OEC) can be discussed, data sources can be aligned on, and expectations can be managed. The last thing you want is to assume your customers want one thing and at the end they’re provided with something completely different.
Risks – numerous risks are associated with data science, and they should all be enumerated as it makes sense for the situation. Risks can span from basic statistical risk, risks associated with business assumptions, or even risks as basic and subjective as not including data or science in the decision-making process.
Regular feedback and update
As stated previously, processes should be in place to alert when sciences start going off the rails. What needs to be decided early, and potentially organizationally, are the frequency of these feedback and update cycles. These may be science-specific but should still consider the end user and the regularity by which improvements are made. Generally, data scientists should automate feedback that will update model parameters freeing up precious resources to focus on more challenging problems.
While there are certainly many others, these four provide a good foundation for opening the dialogue across your organizations. Further, communication must be done early and often to ensure continued alignment with the organization being supported. By openly having this dialogue with your key stakeholders, trust can quickly be established.
Conclusion
As senior leaders continue jumping on the data train, few of them are fully exploiting the potential it can have on their business’s success. Establishing credibility can be one of the most critical components to accelerating these capabilities of your data science organization. While some ownership is in the hands of these key stakeholders, it is ultimately the job of the data scientists to provide quality analyses in a consistent fashion so that trust is established. And at the foundation of credibility is trust.
Visit our knowledge hub
See what you can learn from our latest posts.