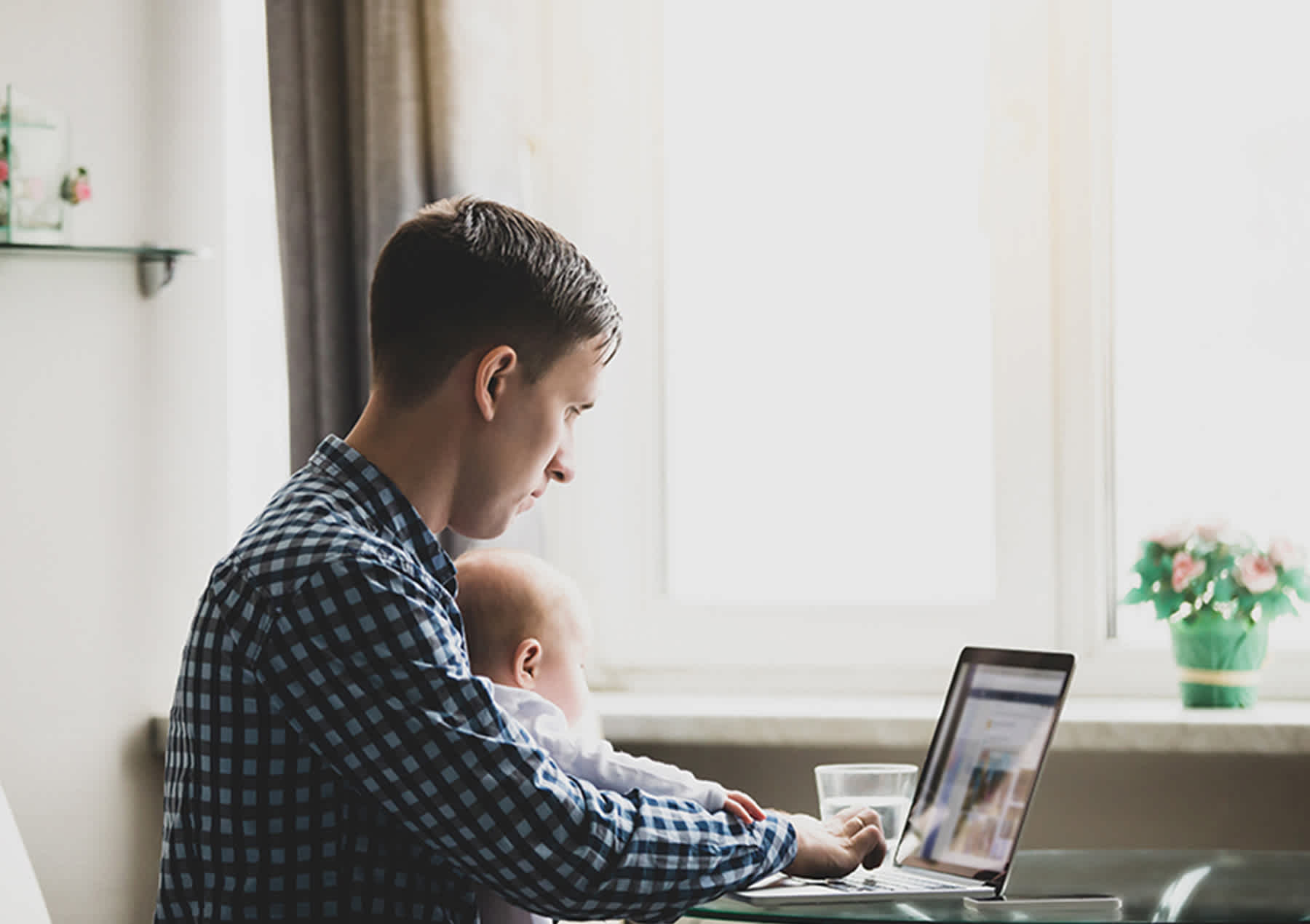
Defining and analyzing the principles of data governance
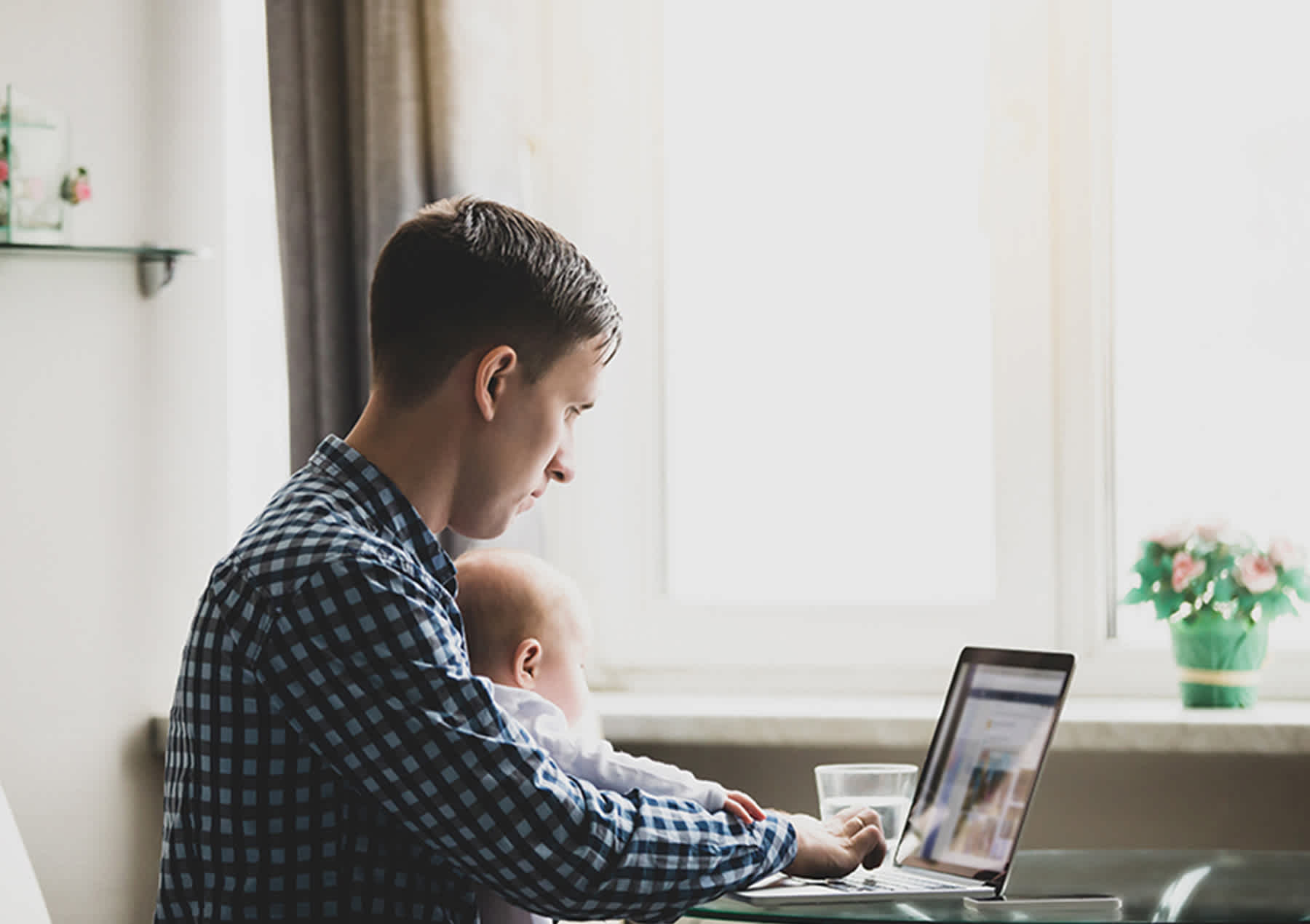
Exponential data growth and regulatory compliance are often cited as catalysts for formal data governance programs. However, the expanding demand and breadth of data science use cases can drive far more complexity than quantity of data alone. These are two different sets of problems with different players involved. Managing data feeds and security is typically the remit of data engineers and DBAs. Harnessing the power of that data for business value, though? That’s the data science wheelhouse.
At 84.51°, we are launching a data governance program centered on science expertise. Our data engineers, architects, and data scientists are collaborating in new ways to understand and shape data on its entire journey from system origin to insight.
Let’s face it. Data governance isn’t the sexiest topic for data scientists. Something about the word governance makes people cringe – let alone “data custodians”. But data governance is about enabling scientists to find, understand, trust, and properly use the ever-growing data assets at their fingertips. It’s about better, faster science.
We rarely quantify those soft, squishy steps before the science can even begin. There are a lot of questions to be answered that aren’t likely to be included in a traditional data catalogue (assuming you have one).
What business logic is already applied to the data source? Are there any store, product, or household exclusions?Do other versions of the data source exist with deviations in business logic?
What upstream processes might affect this data if it is embedded in a process?
Are there any known quality issues with the origin data source?
Where is the source code if I need more info?
… I could go on. At a company with data and science at its core, we aren’t just talking about a single layer of ETL. We have data assets built on models built on science assets built on 84.51° ETL logic, built on Kroger ETL logic .
Finding the answers to these questions currently involves a lot of sifting through email notices and asking around. When I first joined 84.51°, I could typically do a quick lap around our open-office floorplan and get an answer in a few minutes. If it wasn’t clear, then several of us would spontaneously huddle up to discuss and determine next steps. This was the fastest and usually the best way to learn about data origins, definitions, and proper use. The truth is that a whole lot of that information only lived in people’s heads. That works well when you’re a small team that shares the same whiteboard walls – but as well all know, data science is booming and so is 84.51°. While that is a good thing, it makes tribal knowledge inadequate.
We are doing more sophisticated science and applying it to more business areas. There are more teams using similar data in different ways. Without strong governance, conflicts and multiple versions of the truth arise. Without strong data governance, hunting down the reasons for those conflicts feels akin to diving into a black hole of legacy code and vague email questions. “Knowing your data” and all the ways it is used is getting harder.
We have named stewards who oversee both science and data governance. The Science Stewards are responsible for validating the science across domains like Promotions, Pricing, Supply Chain and Targeting. The Data Stewards certify all data. Their task is to ensure data best practices, including making sure the data is discoverable and documented on how it should/should not be used, and verifying that it has a reliable production process that ensures quality and timely delivery, etc.
Visit our knowledge hub
See what you can learn from our latest posts.