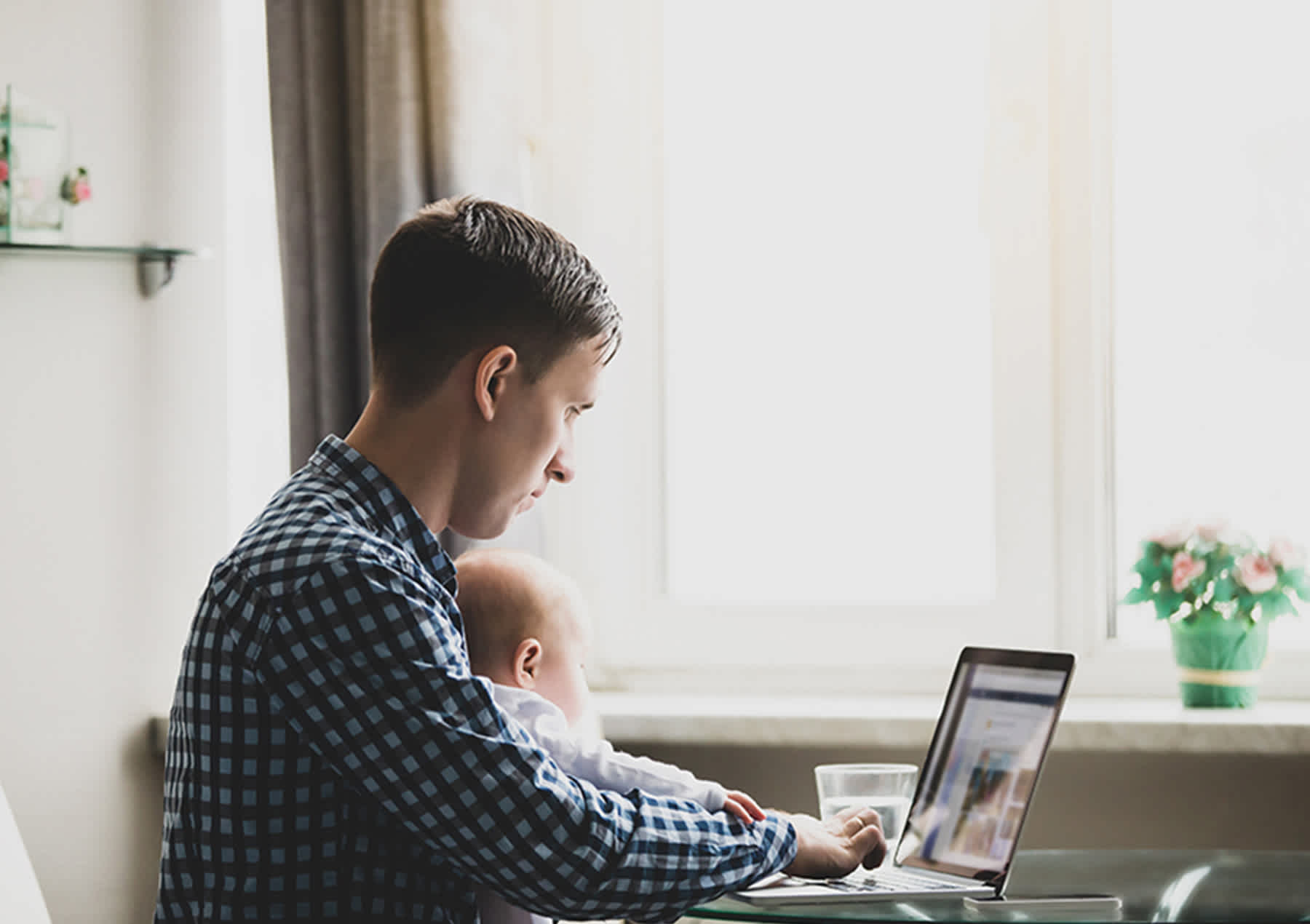
Data Governance for Scientists
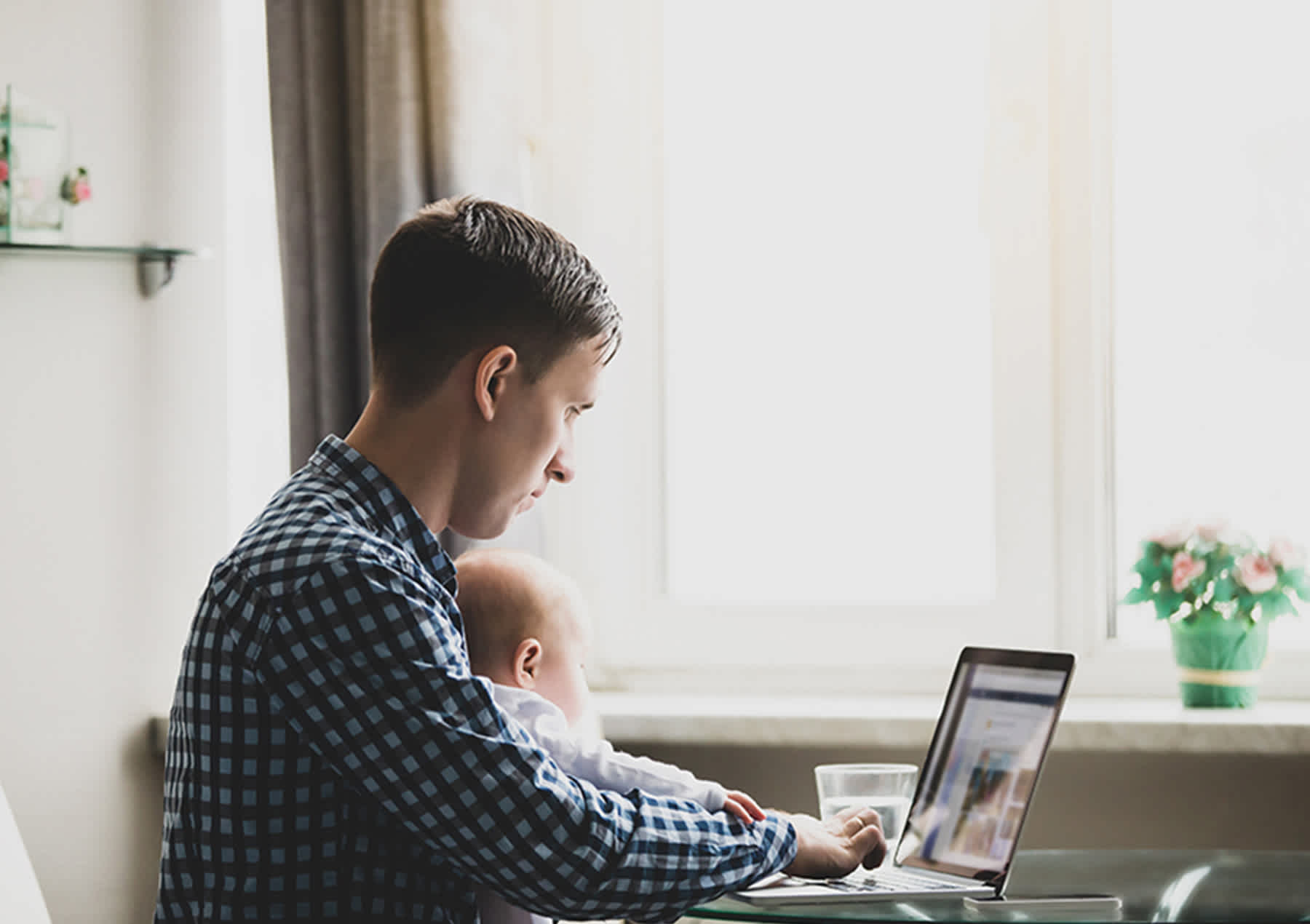
Exponential data growth and regulatory compliance are often cited as catalysts for formal data governance programs. However, the expanding demand and breadth of data science use cases can drive far more complexity than quantity of data alone. These are two different sets of problems with different players involved. Managing data feeds and security is typically the remit of data engineers and DBAs. Harnessing the power of that data for business value, though? That’s the data science wheelhouse.
At 84.51°, we are launching a data governance program centered on science expertise. Our data engineers, architects, and data scientists are collaborating in new ways to understand and shape data on its entire journey from system origin to insight.
Why Data Governance?
Let’s face it. Data governance isn’t the sexiest topic for data scientists. Something about the word governance makes people cringe – let alone “data custodians”. But data governance is about enabling scientists to find, understand, trust, and properly use the ever-growing data assets at their fingertips. It’s about better, faster science.
We rarely quantify those soft, squishy steps before the science can even begin. There are a lot of questions to be answered that aren’t likely to be included in a traditional data catalogue (assuming you have one).
Has anyone done something similar before? What data did they use?
What business logic is already applied to the data source? Are there any store, product, or household exclusions?
Do other versions of the data source exist with deviations in business logic?
What upstream processes might affect this data if it is embedded in a process?
Are there any known quality issues with the origin data source?
Where is the source code if I need more info?
… I could go on. At a company with data and science at its core, we aren’t just talking about a single layer of ETL. We have data assets built on models built on science assets built on 84.51° ETL logic, built on Kroger ETL logic .
Finding the answers to these questions currently involves a lot of sifting through email notices and asking around. When I first joined 84.51°, I could typically do a quick lap around our open-office floorplan and get an answer in a few minutes. If it wasn’t clear, then several of us would spontaneously huddle up to discuss and determine next steps. This was the fastest and usually the best way to learn about data origins, definitions, and proper use. The truth is that a whole lot of that information only lived in people’s heads. That works well when you’re a small team that shares the same whiteboard walls – but as well all know, data science is booming and so is 84.51°. While that is a good thing, it makes tribal knowledge inadequate.
We are doing more sophisticated science and applying it to more business areas. There are more teams using similar data in different ways. Without strong governance, conflicts and multiple versions of the truth arise. Without strong data governance, hunting down the reasons for those conflicts feels akin to diving into a black hole of legacy code and vague email questions. “Knowing your data” and all the ways it is used is getting harder.
Democratize Data, Democratize Code, Democratize Human Expertise
We often hear about “democratizing” data and insights in the industry. Well, there is a big jump between making data available and democratizing sound insights. Data governance gets us there by leveraging technology to scale the code, business understanding, and human expertise that transforms data to insight.
The People
We have named Data Stewards over each science domain who will own the best practices and certification of derived data assets. These are already trusted subject matter experts across domains like Promotions, Pricing, Supply Chain, and Targeting. The technological processes within data governance allow us to democratize their human expertise along with the data itself.
The Technology
Our new data science platform is incorporating a range of data governance requirements to capture information and seamlessly bring it to the user. Data catalogues and system metadata are the two most common elements of data governance. We’re expanding these to cover the information data scientists really need to know for more robust search capabilities.
Science-oriented Metadata Best practice guidelines and business logic in addition to more standard fields like lineage, dates, domain topic tags, and ownership.
Data Source Certification Some data sources are just more reliable than others. A range of certification statuses with column level details enable appropriate trust of the data. Endorsed by Data Stewards and supported through platform workflows.
Reusable Code Components APIs and Domain Function Libraries can capture business logic as parameters and auto-generate metadata. Centrally maintained code drives a single source of the truth.
Search Not only can data scientists search for a data source and find it, they can also find the information they need to properly use it at the same time. Smart search brings the value home.
Slow Down to Speed Up
This harmonious end state doesn’t happen overnight. The technology can scale information, but only for what is included at the point of implementation onward. Most companies have legacy systems and data silos. Different pieces of the puzzle lie in disconnected documentation or in the minds of many different people.
For us, the move to cloud is a critical opportunity to synthesize all of this information. We are already migrating data sources and sciences one-by-one anyway – what better time to capture tribal knowledge and work it into the new tech stack as a foundational element of science workflows?
This can’t happen without full data scientist engagement. As the bridge between data systems and business questions, requirements and metadata look different to us than they do to engineers and DBAs. Consequently, data scientists should be active drivers behind data governance programs. As more of our sciences touch more customers, it’s critical that we understand exactly what the data is in the context of each distinct use case. While this may always be a challenging part of our job, investing in data governance makes it a whole lot easier.
Visit our knowledge hub
See what you can learn from our latest posts.